Harness the
power of data with
Data Science,
Data Analytics &
AI Solutions
Use data science and Artificial Intelligence (AI) to optimise processes, mitigate risk and growing your revenue.
Our team uses data analytics, Business Intelligence (BI) and Machine Learning(ML) to collect, analyse and visualise insights for prediction and decision-making.
Already have a
project in mind?
Our Data Science, Analytics & AI services include

Revenue Analysis and
Optimisation
Identify opportunities with data analytics and AI solutions for revenue growth and increase profitability within a business through data-driven insight instead of assumptions.
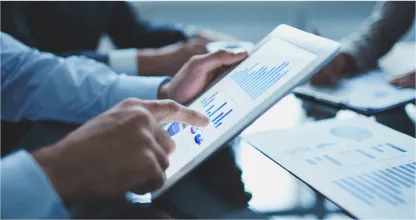

Cost Analysis and
Optimisation
With a thorough assessment of expenses, a business is able to determine inefficiencies, and possible cost reductions and improve the overall financial performance of the business analytics AI, and ML models.
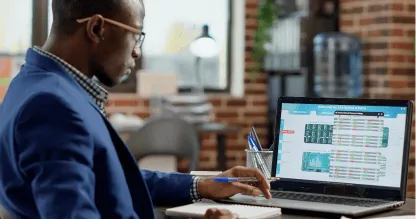

Risk Analysis and
Optimisation
Assess, identify and mitigate risk in various business operations with the use of data science methodologies and implementation strategies.
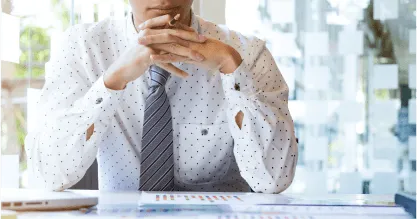

Process Analysis and
Optimisation
Streamline operations and productivity within a business by identifying bottlenecks, inefficiencies, and areas of improvement through data analytics.
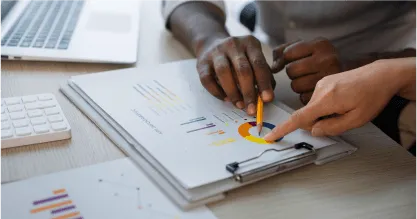

Efficiency Metrics
Quantitative measures are used to gain insight into the efficiency and success of business operations, processes, and resource utilisation. This will assist with performance evaluation, to determine areas of improvement and track goal progress.
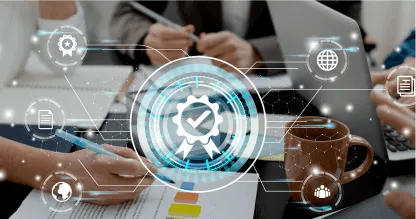

Opportunity Viability
Investigation
Through an opportunity viability investigation, you can assess the potential of a new venture or initiative before allocating recourses that could otherwise be spent more wisely.
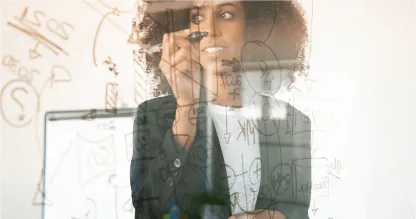
Our Data Science, Analytic
& AI services include
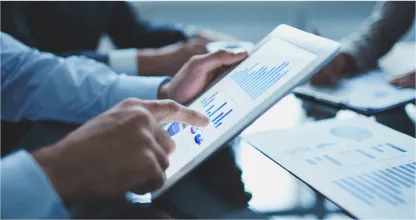
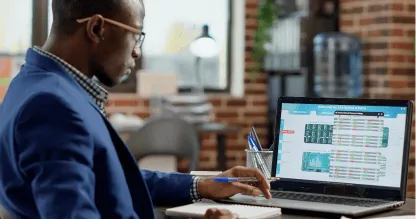
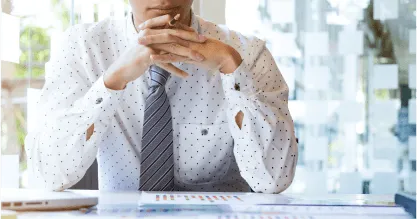
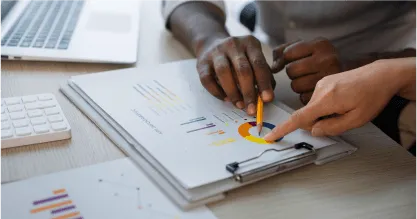
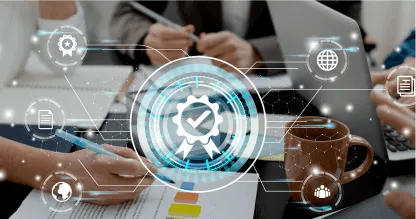
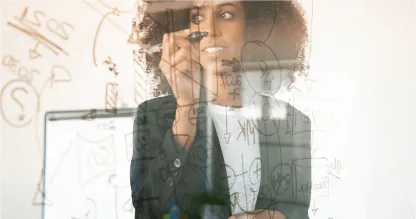
Additional Services
We use Business Intelligence (BI) which is a technology-driven method for data analysis and information delivery that aids managers, employees, and executives in making wise business decisions.
-Dashboards.webp)
Dashboards and Maintenance
Dashboards are designed to visually represent key data in an overview format that is easily consumed and shared. Dashboards are used to monitor progress, gain insight, and track overall performance.
Dashboard Options:
- License-free distributed dashboards for making data widely available.
- Dashboards built in Microsoft Power BI and maintained by our team of experts.
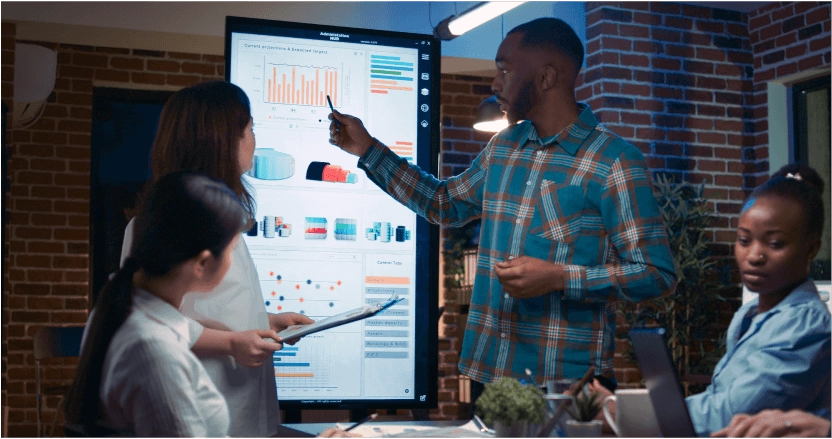
Dashboards are designed to visually represent key data in an overview format that is easily consumed and shared. Dashboards are used to monitor progress, gain insight, and track overall performance.
Dashboard Options:
- License-free distributed dashboards for making data widely available.
- Dashboards built in Microsoft Power BI and maintained by our team of experts.
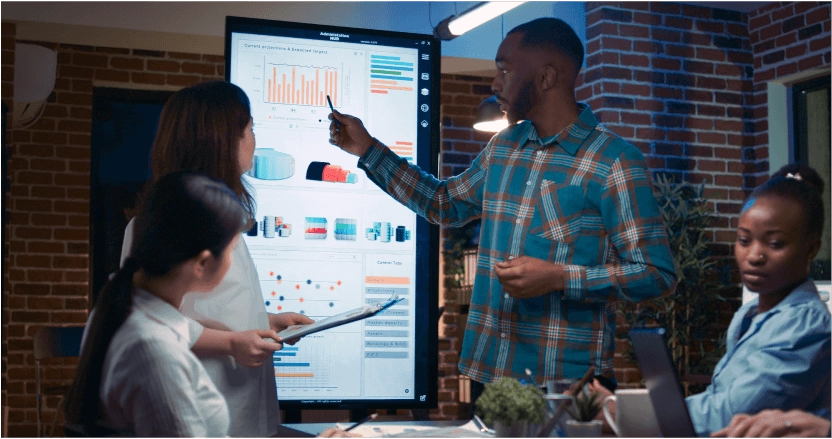
Let’s help you harness
the power of data.
Make informed decisions with our
data science, analytics and AI solutions.
GET STARTED
Benefits of Castigroup Data Solutions
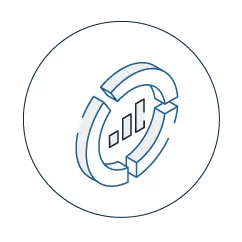
Enhanced Decision-Making
Gain a deeper understanding of operations, customer behaviour and market trends with data analytics, visualisation and process analytics. This enables informed decision-making based on data-driven insights.
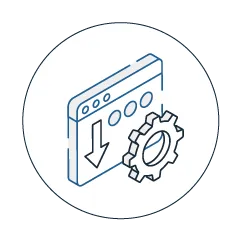
Increased Efficiency
We can help streamline and automate various business processes with data science, AI and ML. This will minimise manual intervention and errors, plus free up valuable resources for more important tasks.
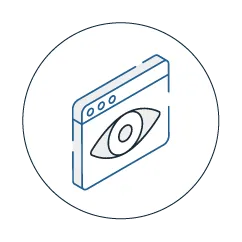
Risk Management
Proactively identify and manage potential risks by using historical data ML and AI models. With data science and analytics, we empower businesses to uncover trends and make informed decisions to minimise adverse outcomes.
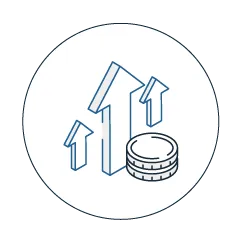
Revenue Generation
Boost revenue with gained insight from sales data analytics, market trends and consumer behaviour. These insights can guide pricing strategies, cross-selling and upselling.
The Benefits of Working with Castigroup

SYNERGY between business & data science teams

FOCUSED on achieving business objectives

DATA-DRIVEN projects for quicker decision making

RAPID PROOF OF CONCEPT (POC) development
Data Science Life Cycle
The Data Science Life Cycle involves a series of essential steps that collectively contribute to effective problem-solving and decision-making through data-driven insights.
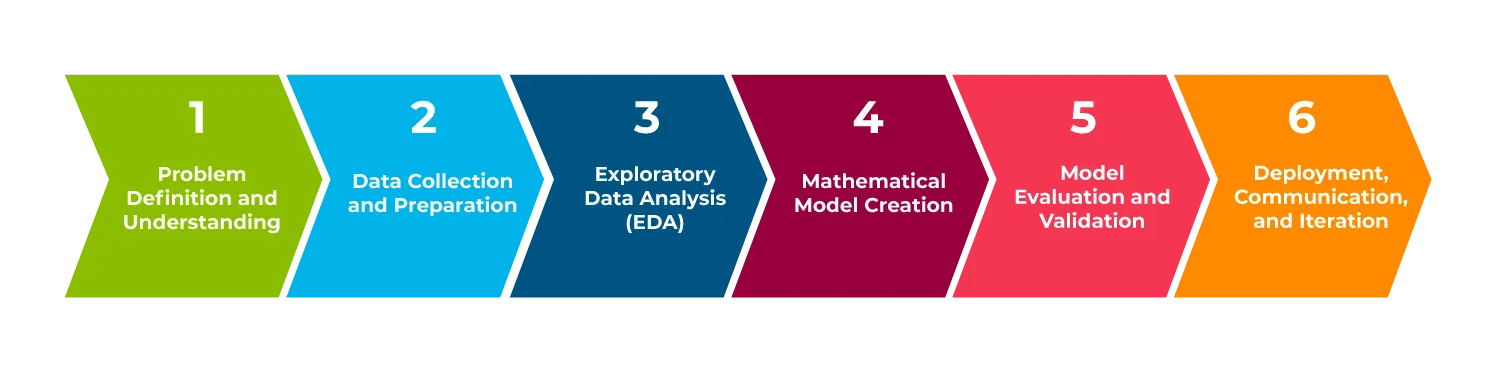
- Feature Engineering and Selection: Features are manipulated and selected to enhance the model's predictive power.
- Model Building and Training: Mathematical algorithms are applied to the data for training, where the model learns patterns and relationships from the data.
Get Started
Let’s start talking about
your data science solutions.